Predicting stock market movements has long been a challenge for investors, economists, and analysts. Traditional financial models, such as the Capital Asset Pricing Model (CAPM) and the Fama-French Three Factor Model, have been widely used for stock return predictions. However, with the rise of machine learning and deep learning, new techniques such as Convolutional Neural Networks (CNNs) are emerging as powerful tools for forecasting stock prices.
In collaboration with Tunis Business School, DevTech Systems conducted a study to determine whether CNNs outperform traditional linear models in predicting stock returns in Saudi Arabia’s Tadawul Stock Exchange. By analyzing data from 2009 to 2022, we compared different forecasting models to assess their accuracy. Our findings show that CNNs consistently outperform linear models, offering investors in emerging markets a more effective tool for making financial decisions.
Why is it so difficult to predict stock prices?
Stock prices are influenced by economic conditions, political events, investor sentiment, and company fundamentals. Traditional linear models assume that stock prices move in predictable patterns, but in reality, financial markets are highly complex and often follow nonlinear trends. These complexities make it difficult for traditional models to capture hidden relationships and unexpected fluctuations in the stock market.
Machine learning techniques, such as deep learning, offer an alternative approach by analyzing historical price data and detecting complex patterns that humans and traditional models may miss. CNNs, originally designed for image processing, have been increasingly applied to financial forecasting due to their ability to extract meaningful features from large, unstructured datasets.
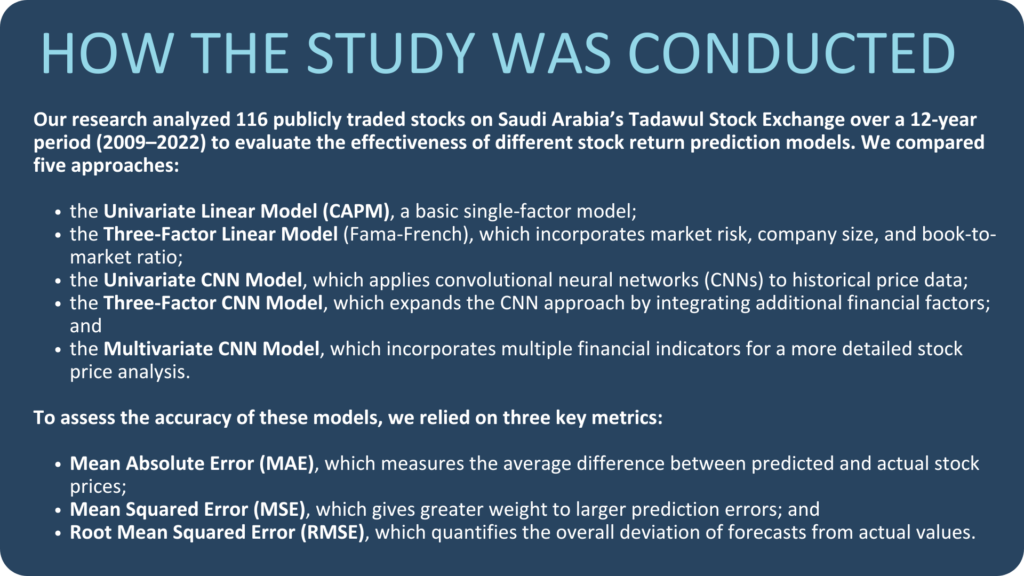
Are CNNs better at predicting stock prices?
Our study found that CNN-based models consistently outperformed traditional linear models across all three accuracy metrics. The univariate CNN model had lower error rates than the CAPM model, while the three-factor CNN model performed better than the Fama-French model. This suggests that CNNs are more effective at identifying complex, nonlinear relationships in stock price movements, which traditional models often fail to capture.
Additionally, our findings show that more data improves prediction accuracy. The multivariate CNN model, which incorporated a broader range of financial indicators – including trading volume, dividend yield, and company profitability—produced the most precise forecasts. This highlights a key strength of deep learning models, which perform better when trained on large, diverse datasets and can detect patterns that conventional models overlook.
Furthermore, CNNs proved particularly effective in emerging markets. Unlike efficient markets, where stock prices often follow rational, well-defined patterns, emerging markets like Saudi Arabia tend to exhibit inefficiencies, meaning prices can be influenced by factors that traditional models fail to capture. Our research found that CNN models were better at identifying and exploiting these inefficiencies, making them an especially valuable tool for investors looking to navigate and capitalize on the unique characteristics of emerging markets.
What does this mean for investors and policy makers?
For investors and financial analysts, integrating AI and deep learning into stock selection and forecasting models offers a more data-driven and adaptive approach to decision-making. Traditional models like the CAPM and Fama-French Three Factor Models rely on linear assumptions that often fail to capture the complex nature of stock price movements, especially in volatile and emerging markets. In contrast, CNNs can analyze large datasets, detect nonlinear relationships, and identify hidden patterns, giving investors and analysts a competitive edge. This allows for more accurate risk assessment, improved asset allocation, and enhanced portfolio diversification. AI can also incorporate alternative data sources, such as trading volume, sentiment analysis, and macroeconomic indicators, providing a richer and more comprehensive view of the market. As deep learning models become more widely adopted, financial professionals who leverage these tools will be better equipped to navigate uncertainty and develop more effective investment strategies.
For policymakers, AI presents a new way to assess market efficiency and detect potential distortions in financial markets. The Efficient Market Hypothesis (EMH) classifies markets into three types: weak-form efficiency, where stock prices reflect all past market data; semi-strong efficiency, where prices incorporate all publicly available information; and strong-form efficiency, where all information, including insider knowledge, is fully reflected in prices. Traditional models often struggle to determine whether markets adhere to these efficiency levels, but AI can analyze patterns in stock price movements, trading behavior, and market reactions to news events to reveal inefficiencies. By using CNNs and other deep learning models, regulators can identify anomalies, pricing distortions, speculative bubbles, and abnormal volatilities that signal market inefficiencies. This insight will enable policymakers to adjust regulations, improve market transparency, and implement corrective measures to enhance financial stability. Additionally, as AI-driven trading strategies become more widespread, regulators may need to monitor algorithmic trading, prevent market manipulation, and adapt policies to ensure fair competition.